What is AI Review?
1.1 Workflow
FRONTEO’s AI Review workflow harnesses the power of AI throughout the review workflow to deliver transparency, defensibility, and efficiency – with built-in validation checks. AI Review is the next step in the Technology Assisted Review (TAR) arena, which is already widely accepted in eDiscovery. Many review platforms offer some kind of TAR, and some US government agencies use TAR and actively encourage its use.
FRONTEO calls its unique combination of technology and AI-driven workflow KIBIT Automator, or KAM. FRONTEO aims to make every stage of the AI Review process more efficient and effective through AI. FRONTEO calls its unique combination of technology and AI-driven workflow KIBIT Automator, or KAM. FRONTEO aims to make every stage of the AI Review process more efficient and effective through AI and believes eDiscovery in AI will be a big part of the future of the work of legal professionals.
FRONTEO's adoption of Technology-Assisted Review through the KIBIT Automator marks a significant leap forward in legal document analysis. This approach enhances the precision and speed of document review, setting new industry standards. By integrating TAR with advanced AI, FRONTEO is at the forefront of a transformative shift towards streamlined legal reviews, underscoring our commitment to technological excellence in legal outcomes.
Now, let's delve deeper into how FRONTEO's AI Review workflow revolutionizes the traditional review process to maximize transparency and defensibility.
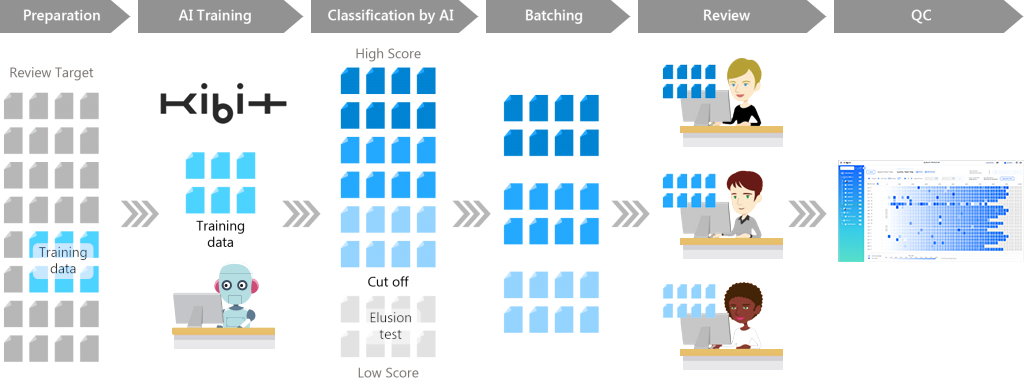
Figure 1: AI Review Process
Figure 1 above illustrates the AI Review workflow. First, the review target is established. The entire document corpus can be designated for review, or the review target can be narrowed. This narrowing is frequently accomplished through a keyword search, but in some cases, TAR (such as Concept Analysis) is used to narrow the review target.
Next, the AI engine, KIBIT, is trained. FRONTEO samples the review target and a small amount is reviewed and coded to make the KIBIT training data set. After KIBIT is trained with the training data, KIBIT’s training model is evaluated by typical statistical indicators such as Recall, Precision, and Elusion rate. Once the client/law firm agrees that KIBIT’s statistical indicators are acceptable, the KIBIT training model is applied to the entire review target.
KIBIT scores each document based on an assessment of how likely the document is responsive on a scale of 10,000 down to 0 (Relevancy Score). Relevancy Scoring can be used for two things. First, documents can be batched to reviewers by KIBIT based on the similarity of relevancy scoring – meaning similar documents can be reviewed together. The difference between KIBIT and a typical similarity search is that KIBIT calculates the relevance score by analyzing the context and value of the document, not the superficial structure (similar sentences, words, etc.). Second, a “cut off” can be established whereby documents below a certain score will be deemed non-responsive, and not be reviewed. The cut-off point can be determined on a case-by-case basis, based on the statistical model associated with that case. Review resources are then focused on documents most likely to contain responsive documents.
To confirm that the cut-off point selected is appropriate, an Elusion test is performed on a sample of the cut-off documents to confirm these are non-responsive. A statistical model is used to help assess whether the frequency of any responsive documents found below the cut off is reasonable.
FRONTEO also offers a truly unique AI-driven tool within its AI Review for quality control (QC) of the documents subject to manual review: a “QC Heat Map.” In this AI-driven feature, the results of human reviewers are measured against the predicted classification of the KIBIT score, and the results of the QC can be used to evaluate the efficacy and quality of the human reviewer. Thus, the QC Heat Map and the use of feedback to improve batching to reviewers brings AI capability into every stage of the AI Review process.
Compared to linear review, AI Review reduces the number of documents that must be reviewed by humans, increases review speed by reorganizing documents in order of responsiveness and by assigning batches based on relevancy scoring, and improves review quality through implementing AI within the QC process.
Figure 1 above illustrates the AI Review workflow. First, the review target is established. The entire document corpus can be designated for review, or the review target can be narrowed. This narrowing is frequently accomplished through a keyword search, but in some cases, TAR (such as Concept Analysis) is used to narrow the review target.
Next, the AI engine, KIBIT, is trained. FRONTEO samples the review target and a small amount is reviewed and coded to make the KIBIT training data set. After KIBIT is trained with the training data, KIBIT’s training model is evaluated by typical statistical indicators such as Recall, Precision, and Elusion rate. Once the client/law firm agrees that KIBIT’s statistical indicators are acceptable, the KIBIT training model is applied to the entire review target.
KIBIT scores each document based on an assessment of how likely the document is responsive on a scale of 10,000 down to 0 (Relevancy Score). Relevancy Scoring can be used for two things. First, documents can be batched to reviewers by KIBIT based on the similarity of relevancy scoring – meaning similar documents can be reviewed together. The difference between KIBIT and a typical similarity search is that KIBIT calculates the relevance score by analyzing the context and value of the document, not the superficial structure (similar sentences, words, etc.). Second, a “cut off” can be established whereby documents below a certain score will be deemed non-responsive, and not be reviewed. The cut-off point can be determined on a case-by-case basis, based on the statistical model associated with that case. Review resources are then focused on documents most likely to contain responsive documents.
To confirm that the cut-off point selected is appropriate, an Elusion test is performed on a sample of the cut-off documents to confirm these are non-responsive. A statistical model is used to help assess whether the frequency of any responsive documents found below the cut off is reasonable.
FRONTEO also offers a truly unique AI-driven tool within its AI Review for quality control (QC) of the documents subject to manual review: a “QC Heat Map.” In this AI-driven feature, the results of human reviewers are measured against the predicted classification of the KIBIT score, and the results of the QC can be used to evaluate the efficacy and quality of the human reviewer. Thus, the QC Heat Map and the use of feedback to improve batching to reviewers brings AI capability into every stage of the AI Review process.
Compared to linear review, AI Review reduces the number of documents that must be reviewed by humans, increases review speed by reorganizing documents in order of responsiveness and by assigning batches based on relevancy scoring, and improves review quality through implementing AI within the QC process.
1.2 AI Review Increases Review Speed
At FRONTEO, we know from experience that review speed increases when reviewers review similar documents together. Thus, for AI Review, we use KIBIT’s Relevancy Score to create high scoring batches and low scoring batches.
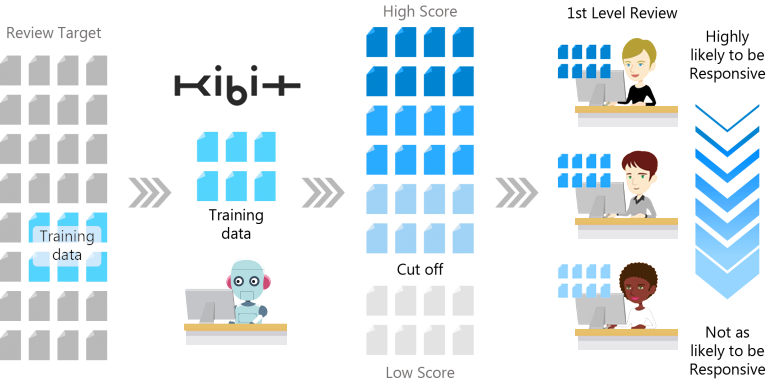
Figure 2: Assign batches to reviewers based on KIBIT’s Score
For high scoring batches, due to the high number of responsive documents, we have found review speed to be slow, on average 20 documents/hr. In contrast, low scoring batches contain a greater proportion of non-responsive documents and have a faster review speed, of around 60 documents/hr and sometimes higher. Figure 3 illustrates the change in review speed due to scored batching within AI Review using KAM compared to linear review.
For high scoring batches, due to the high number of responsive documents, we have found review speed to be slow, on average 20 documents/hr. In contrast, low scoring batches contain a greater proportion of non-responsive documents and have a faster review speed, of around 60 documents/hr and sometimes higher. Figure 3 illustrates the change in review speed due to scored batching within AI Review using KAM compared to linear review.
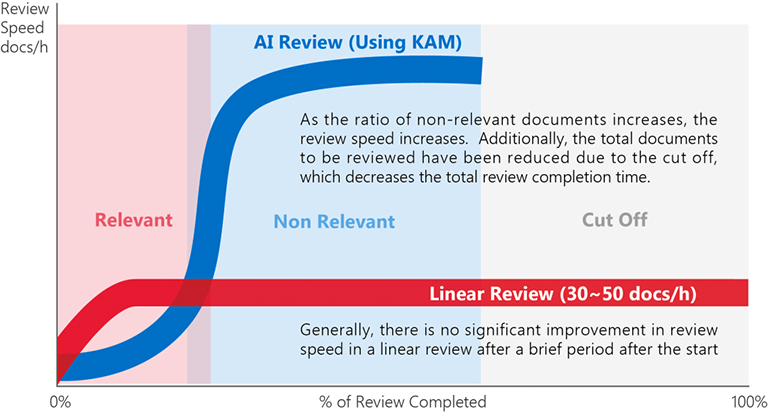
Figure 3: Review Speed: AI Review vs. Linear Review
1.3 Visualized QC (Heat Map)
The QC Heat Map compares the AI-modeled prediction assigned to a document against the human result (a “before and after”) and presents these visually by color to empower the Review Manager to identify where the human reviewers and computer disagree about the classification or coding of a document. QC can be efficiently and effectively managed with the assistance of this technology.
Each individual box represents a set of documents within a range of KIBIT relevancy scores. The box color is determined by the percentage of documents a reviewer has coded relevant. If most or all of the documents are coded non-relevant, then the box is green. If most or all of the documents are coded relevant, then the box is red. If the reviewer has coded likely non-relevant documents as non-relevant and likely relevant documents as relevant, the reviewer’s coding on the heat map will look like a clean gradient from white to green to red.

Figure 4: Heat Map Gradient Bar
When the reviewer’s coding does not match the gradient, the QC Heat Map will reveal this, and create a visual signal to check those documents to see if there are any coding mistakes. See the below heat map as an example.
When the reviewer’s coding does not match the gradient, the QC Heat Map will reveal this, and create a visual signal to check those documents to see if there are any coding mistakes. See the below heat map as an example.
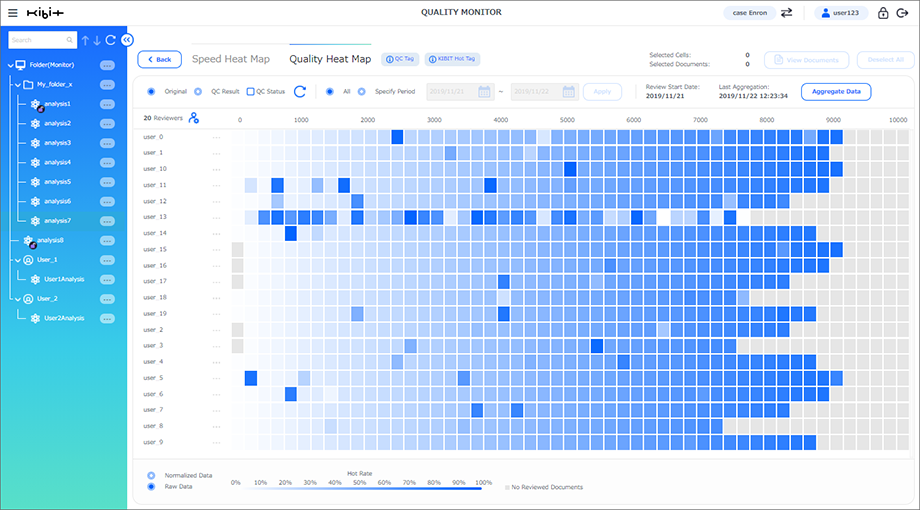
Figure 5: QC Heat Map
In this QC Heat Map, Reviewer 6 has coded several documents with a high relevancy score as not relevant. In contrast, Reviewer 15 has coded several documents with a low relevancy score as relevant. The QC Heat Map also shows at a glance that the coding of these two reviewers is different from all other reviewers, which increases the likelihood that this discrepancy is due to a coding mistake likely arising from a misinterpretation of documents or a misunderstanding of review instructions.
The QC Heat Map is a way to capture an overall view of the quality of reviewer coding. Using the QC Heat Map, you can identify potential coding problems in a way that could not be captured through traditional keyword or tag searches used in most review QC checks.
In this QC Heat Map, Reviewer 6 has coded several documents with a high relevancy score as not relevant. In contrast, Reviewer 15 has coded several documents with a low relevancy score as relevant. The QC Heat Map also shows at a glance that the coding of these two reviewers is different from all other reviewers, which increases the likelihood that this discrepancy is due to a coding mistake likely arising from a misinterpretation of documents or a misunderstanding of review instructions.
The QC Heat Map is a way to capture an overall view of the quality of reviewer coding. Using the QC Heat Map, you can identify potential coding problems in a way that could not be captured through traditional keyword or tag searches used in most review QC checks.
Method for Evaluating KAM’s Effectiveness
To evaluate KAM’s effectiveness for speed of review in terms of eDiscovery and artificial intelligence, we simulated a standard linear review using publicly available Enron data, a review protocol, and an actual team of reviewers managed by an attorney. We utilized the results of this simulated review to estimate the increased effectiveness of the AI Review.
We simulated a linear review using data from the 2000 Enron case, actual reviewers, and a review protocol. We performed a keyword search to create a review target. The review target had 9,681 documents. Our team of six 1st level reviewers took a total of 6 days (261 hours) to review the review target of 9,681 documents. The average review speed was 37 documents/hr, and the review target had a richness of 4.8%.
We used the coded documents from the mock linear review to simulate KAM’s performance. We used 365 coded documents as training data. We found that, if we had used an AI Review process with KAM, we could have eliminated 68.9% of the review target (6,668 documents out of 9,681) with a Recall rate of 86.67%, an Elusion Rate of 2.98%, leaving only 3,013 documents to review. We determined (based on prior experience) the rates to be acceptable.
Based on historical data, we know that generally high score batches have a review rate of 20 documents/hr. while low score batches are reviewed at 60 documents/hr. We estimated the number of higher scoring (likely relevant) documents based on a 5% richness versus the number of documents to be reviewed.
Thus, we estimate that, compared to the actual linear review we conducted, if we had used KAM and the KIBIT technology, we could have completed review of the overall review target (including cut off documents) in 55 hours, at a rate of 177 documents/hr. Even excluding the cut off documents, we estimate the review rate would have be approximately 55 documents/hr, still much faster than linear review.
These results demonstrate that leveraging strategic eDiscovery and AI technology provides a significant improvement in the efficiency of document review processes. This groundbreaking technology allows for the identification of relevant documents more quickly and accurately than ever before.
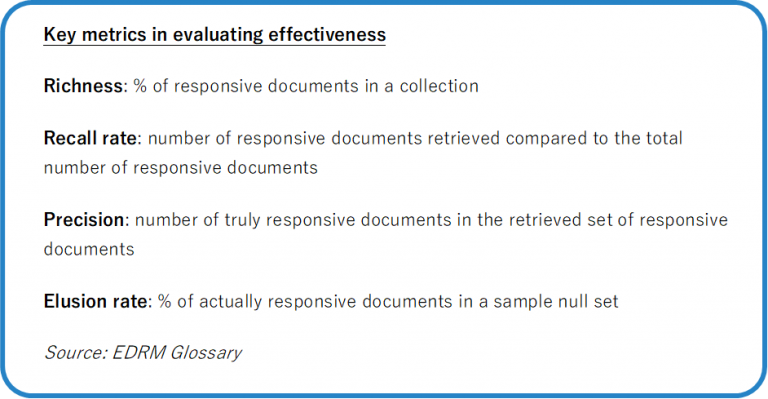
KAM in a Real Case
KAM has also been used in real cases to cut off and prioritize documents. In a very recent case with a tight deadline, the review target was approximately 30,000 documents collected from 10 custodians. Using 380 coded documents as KIBIT training data, we were able to eliminate 13,500 from the review (45% of the overall review target), with an Elusion rate of 5.5%. Thus, reviewers only reviewed 16,500 documents out of the original 30,000 documents. As a result, the overall review speed, including cut-off documents, was 80 documents/hr.
Conclusion
KAM has proven to be – in both mock reviews and actual matters – a game-changing way to incorporate AI into the entire review workflow. FRONTEO has established that KAM is highly effective and requires very few documents to develop a reliable training model for eDiscovery in AI. KIBIT creates and validates a defensible cut-off point, thereby greatly reducing the number of documents requiring manual review. Even the manual review portion is more effective due to the document prioritization batching based on relevancy scoring and the use of AI within the review QC process (QC Heat Map). We estimate that the overall review speed in an AI review is nearly twice as fast as a standard linear review. And, the QC Heat Map can support a comprehensive and more accurate QC of the linear review process. Overall, AI Review with KAM achieves dramatically increased review speeds while maintaining – or improving – review quality.
In a world where litigation and legal matters move faster than ever, the use of AI in eDiscoveryshould be explored as a way for firms to streamline thedocument review process. FRONTEO is already at the forefront of using Technology-Assisted Review and AI technology in doc review. We use our own proprietary tools and innovative new tools to empower lawyers and other clients dealing with mountains of evidence from challenging legal cases and projects.
Do you have questions about the power of eDiscovery and AI? We have answers.
FRONTEO’s Data scientists and Review experts are standing by to answer your questions.